MEET THE ARTISTS: Uncharted Limbo Collective
An experiment in human-AI collaboration
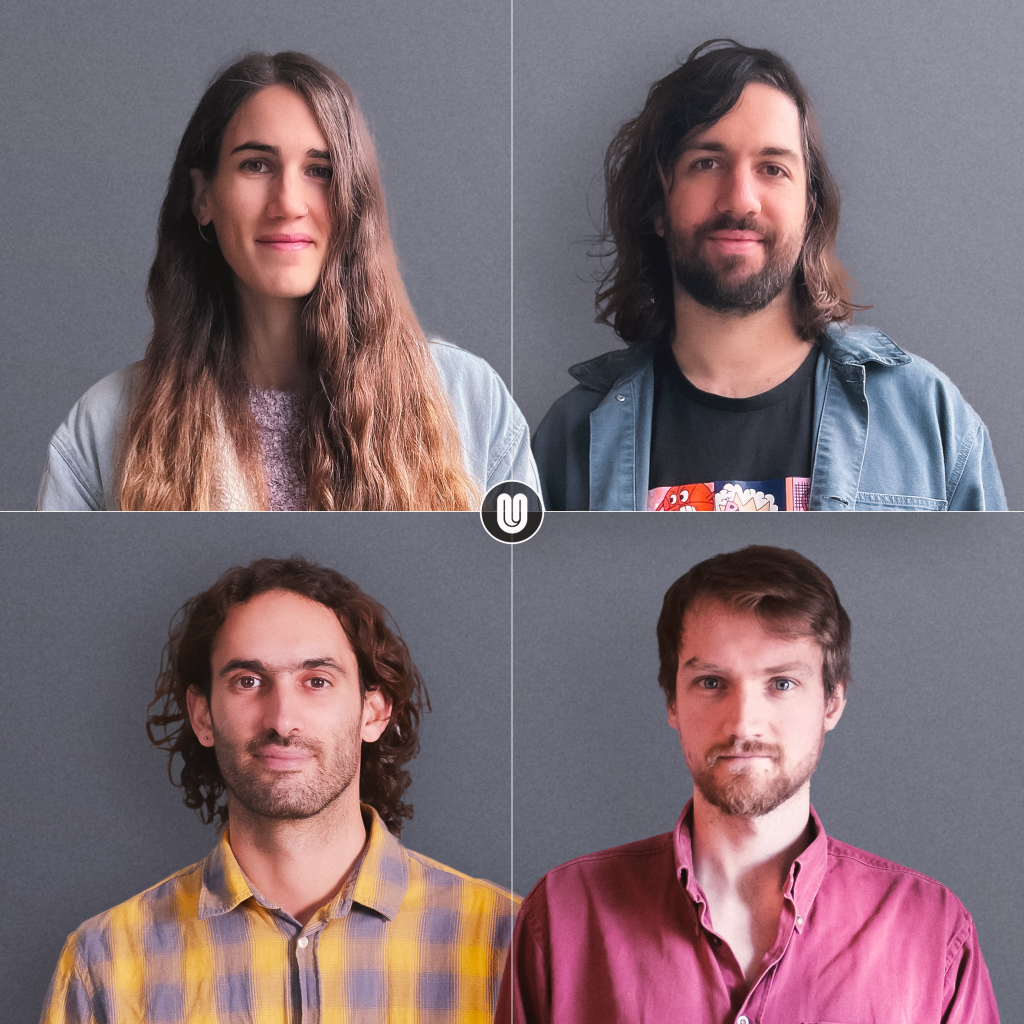
Uncharted Limbo Collective is a team of creative coders and visual artists based in Athens and London who share a background in architecture but have expanded to develop creative projects using state-of-the-art digital tools. In their project Monolith, they will explore methods for facilitating communication and collaboration between humans and what they characterize as “digital beings.”
At HLRS, Uncharted Limbo will conduct experiments with dancers and movement artists. An algorithm they are developing will generate an audiovisual environment in HLRS’s CAVE visualization facility to prompt the dancers’ motions. At the same time, they will use sensors to capture data characterizing the dancers’ movements and interior states such as heart rate and neural oscillations. These data will then be analyzed using deep neural networks, and the results will be fed back into the algorithm to train it to recognize and respond to patterns in the dancers’ movements. During the development of this method, Uncharted Limbo also plans to work with S+T+ARTS AIR partner Sony Computer Science Laboratories, who conduct research on augmented creativity. The team expects that by gathering and analyzing many hours of data, the algorithm should learn to anticipate, react to, and encourage human movements and emotions in an increasingly confident and deliberate way.
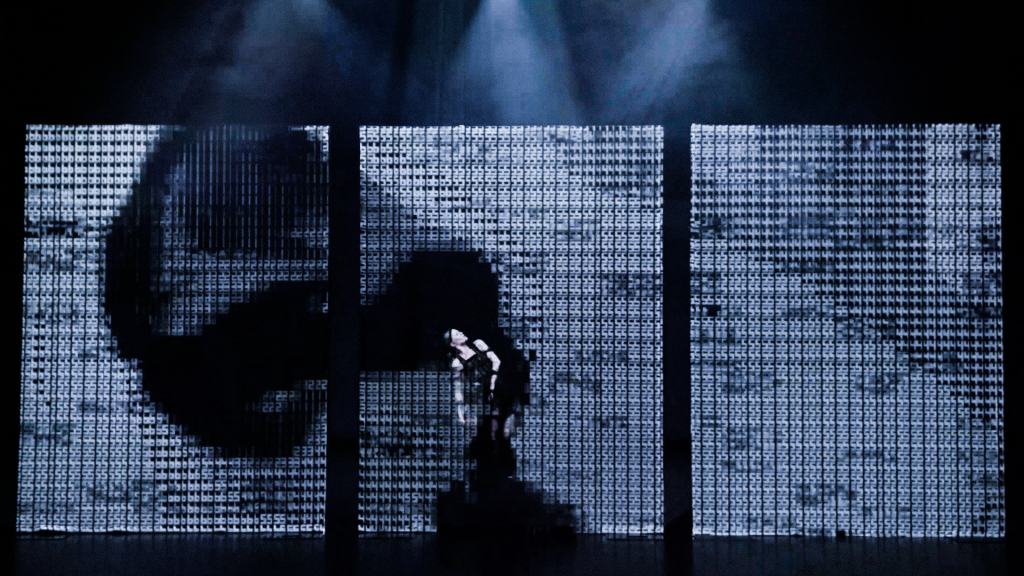
At the conclusion of the project, the team will stage a performance involving equal cooperation between one or more dancers and the resulting digital being — humans will react to audiovisual impulses while the algorithm responds to their movements, creating a performative dialogue between humans and a machine. In addition to testing new interactive technologies, Uncharted Limbo will use the project as a way to engage the public in thinking about issues related to artificial agency, digital presence, data ownership, and the omnipresence of digital technology. The results will also be available to be exhibited elsewhere in the future.
“S+T+ARTS Air offers us a unique opportunity to develop a project we’ve been discussing for a while — an urge to address the important questions of agency and emergent sentience of digital entities,” commented Eleana Polychronaki on behalf of Uncharted Limbo. “As artists with significant experience in coding and art-directing real-time visuals for dance performances and interactive installations, we found ourselves wondering how we can break the norm of the typical ‘accompanying visuals’ and ‘one-way reactivity’ seen in most New Media performances. We hope that by collaborating with the HLRS team and taking advantage of their expertise in innovative visualization and access to supercomputing power we will be able to contribute to the immense field of research that explores human-machine interaction and the ever-evolving dynamics of that relationship.”
Reflection and thoughts on the project by Uncharted Limbo Collective:
- Significant progress has been made in developing real-time camera-based motion tracking solutions, which are crucial for training our neural networks (NN) effectively and for future applications (generative visuals and audio systems).
- These advancements allow the system to capture motion data in real-time, enhancing the quality and accuracy of the datasets used for training our machine learning models.
Machine Interpretation:
- Data extraction from archive mocap sequences: Existing motion capture sequences from movement workshops have been processed, allowing the system to leverage a more diverse range of motion data. This data contributes to a richer training dataset for our AI models.
- Data formatting to use for AI training (remove bias): Careful data formatting has been undertaken to ensure that the training dataset is free from biases, thereby improving the fairness and accuracy of the model. By removing redundancies and ensuring balanced representation, the model can generalise better to different types of motion inputs.
- Motion Capture Classifier custom model [LSTM Architecture]: A custom Long Short-Term Memory (LSTM) architecture has been developed to classify motion capture data. LSTMs are well-suited for this task due to their ability to handle temporal sequences, allowing for more nuanced understanding and prediction of motion patterns.
- Post-Process class clustering from PaCMAP dimension reduction: After training, the Pairwise Controlled Manifold Approximation (PaCMAP) technique is employed to reduce the high-dimensional LSTM output into a more manageable form. This allows for effective visualisation and clustering of motion classes, enabling easier identification of patterns and relationships within the data.
Inside the Machine Brain:
- Spatial visualisation of LSTM latent space: The LSTM’s latent space is visualised in an almost gamified manner to intuitively understand how the model internally categorises different types of motion. This provides insights into how the machine thinks, learns and critically perceives similarities or differences between various motion sequences (displaying these as neighbours with spatial similarities).
Collaboration with AIR partners:
- Continued useful discussions with the HLRS team, receiving consistent feedback on the overall project and the ‘work in progress visualisation system.’
- Shared progress on motion capture development, though HLRS’s expertise in this area is limited, resulting in less crossover.
- Collaboratively explored potential features and applications for other datasets or fields.
- Shared experiences with other artists, particularly with Natan Sinigaglia, to consolidate ideas through discussions about his motion classification project.
- Attended the HLRS ReACH II Workshop in Stuttgart in September, where an in-depth presentation and Q&A session allowed discussion of findings and invited new perspectives and routes of enquiry.
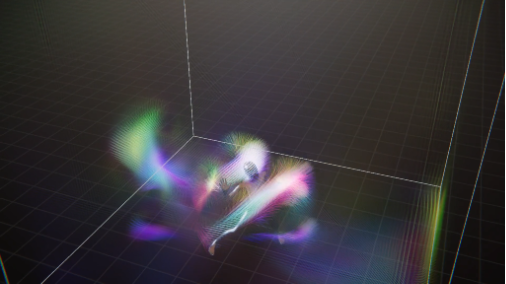
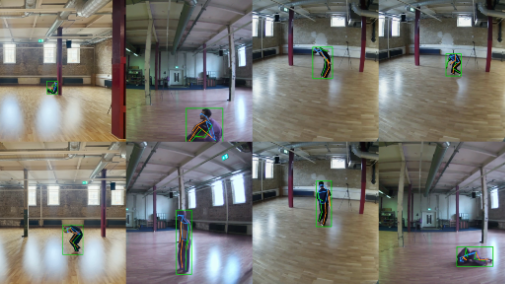
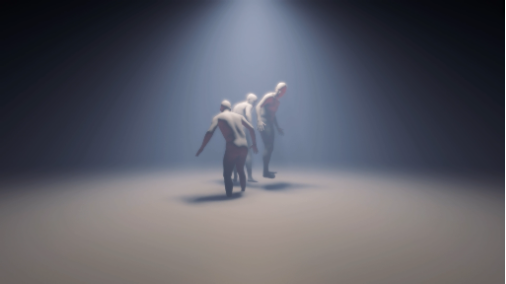
KNOWLEDGE TRANSFER
The first knowledge transfer session of Uncharted Limbo Collective was a public presentation at the second 2024 iteration of the ReACH workshop, organized by MSC and HLRS. The workshop was visited by artists, researchers and policy experts. The presenting artist George Adamopoulos guided a nearly two-hours-long session, presenting the Monolith’s artistic concept and methodology as well as their journey of technological exploration. A prototype of the Latent Spaceship tool was presented presented in a live demo. The session was concluded by a Q&A with the audience.
Event 1 Details
- Event Name: ReACH Workshop (Research and Creation Center for eCulture and the Humanities)
- Date: September 25-27, 2024
- Location: High-Performance Computing Center Stuttgart (HLRS)
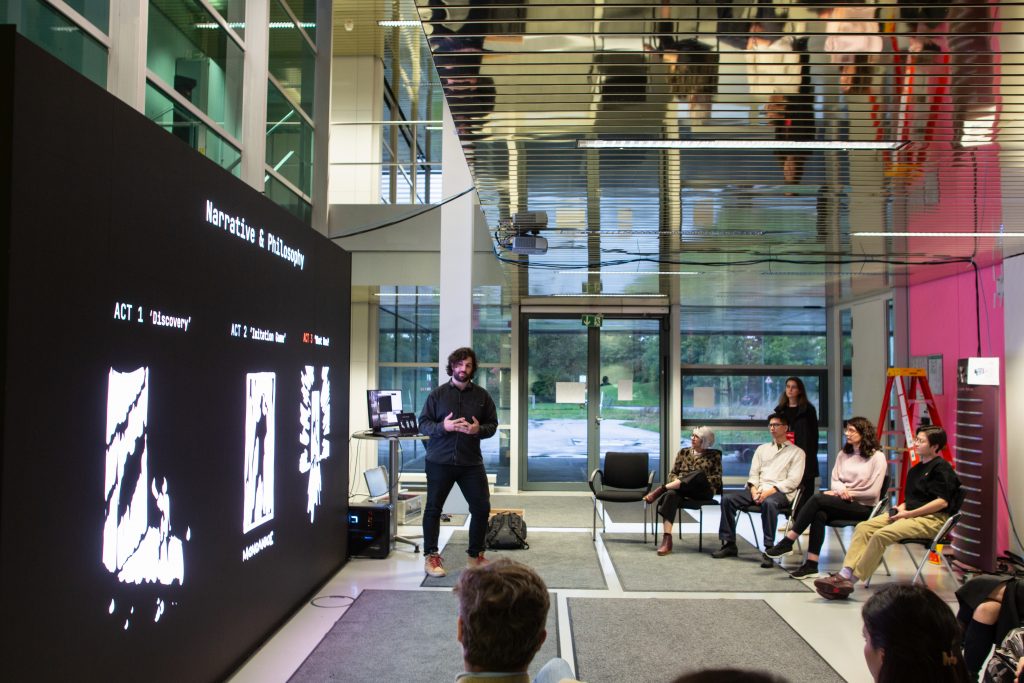
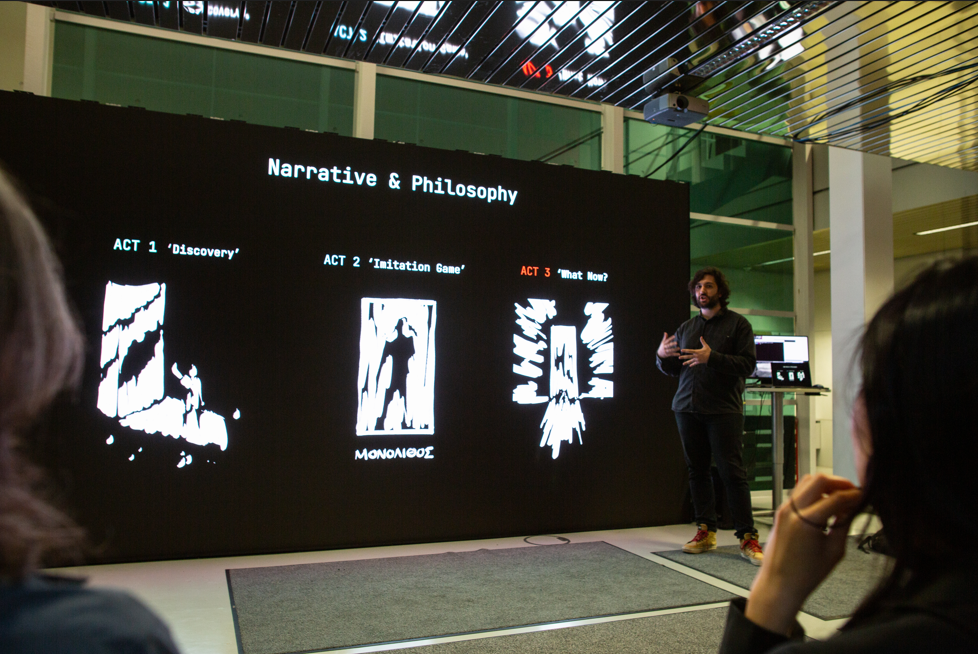
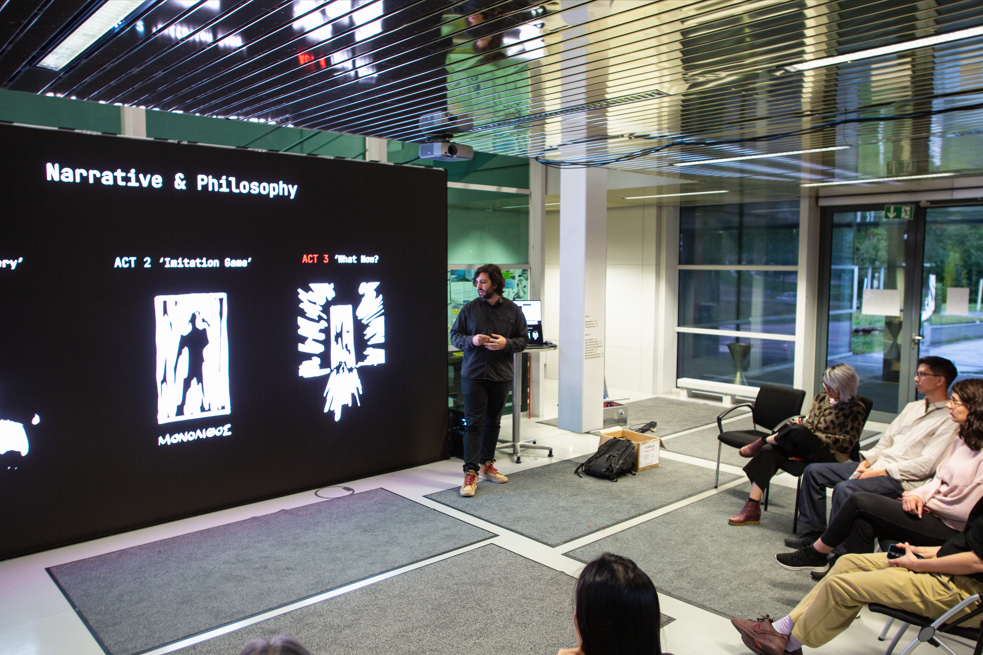
Event 2 Details
For their second knowledge transfer session, the collective took part in a special dedicated event called “Motion Prompting,” which brought together diverse perspectives from artists, technologists, researchers, and industry leaders. This event explored the frontier where AI meets human movement, addressing crucial questions about how artificial intelligence can interpret and respond to physical expression. Through the presentation of the Monolith project participants examined the current capabilities and future potential of motion prompting technology. The event sparked discussions about moving beyond text-based AI interactions, investigating how neural networks perceive the human body, and exploring methods for AI to analyze movement quality.
- Event Name: ReACH Workshop (Research and Creation Center for eCulture and the Humanities)
- Date: September 25-27, 2024
- Location: High-Performance Computing Center Stuttgart (HLRS)
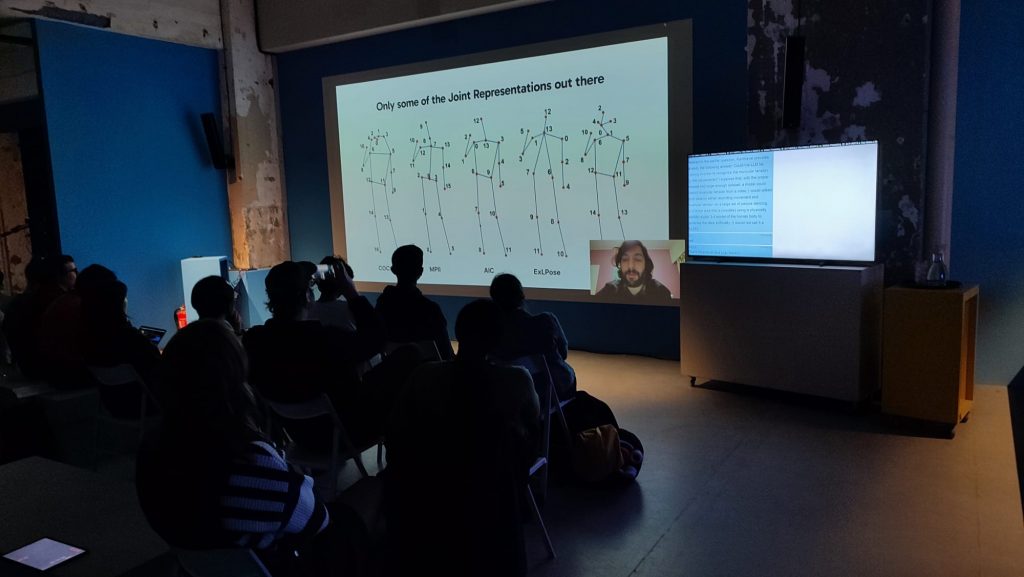

This project is funded by the European Union from call CNECT/2022/3482066 – Art and the digital: Unleashing creativity for European industry, regions, and society under grant agreement LC-01984767